Cryptocurrency prediction, the Power of Machine Learning
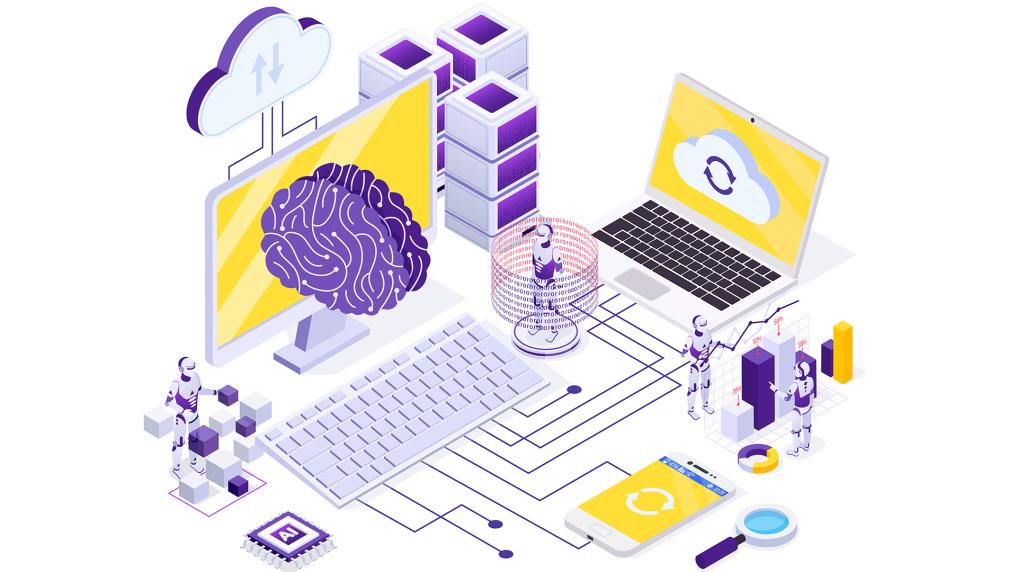
Cryptocurrency prediction has become an increasingly relevant field in the financial industry, where investors and traders seek to gain insights into the future performance of digital assets. With the volatility and complexity of cryptocurrency markets, traditional methods of analysis often fall short in accurately predicting price movements. This is where the power of machine learning comes into play, stay with Forvest.
Machine learning algorithms have demonstrated their efficacy in handling large volumes of data and identifying patterns that are not easily discernible to human analysts. In the context of cryptocurrency prediction, machine learning leverages historical price data, trading volumes, market sentiment, and various other factors to generate predictive models.
To understand cryptocurrency prediction through machine learning, it is essential to grasp the concept of training models. Machine learning models are trained using historical data, where they learn patterns and relationships between input variables (such as past price trends, trading volumes, and news sentiment) and corresponding output variables (future price movements). These models then use these learned patterns to make predictions on unseen data.
Introduction to Machine Learning in Cryptocurrency Analysis
Machine learning has emerged as a powerful tool in the realm of cryptocurrency analysis. With the ever-growing complexity and vast amount of data generated by cryptocurrency markets, traditional analysis techniques often fall short in providing accurate and timely insights. Machine learning, on the other hand, enables the development of models that can effectively process and interpret this data.
In cryptocurrency analysis, machine learning algorithms can be trained to recognize and learn patterns from historical market data, including price movements, trading volumes, and market sentiment. These algorithms can then utilize this learned knowledge to make predictions and identify trends in the market. By leveraging the capabilities of machine learning, analysts and investors can gain valuable insights into market dynamics, identify potential investment opportunities, and mitigate risks in the highly volatile cryptocurrency landscape. As machine learning continues to advance, it is expected to play an increasingly pivotal role in analyzing and understanding the intricacies of cryptocurrency markets.
The Role of Historical Data in Cryptocurrency Prediction
The role of historical data in cryptocurrency prediction cannot be understated. Historical data serves as a vital foundation for training machine learning models and enabling them to make accurate predictions. By analyzing past price movements, trading volumes, and market trends, these models can identify patterns and relationships that can inform future predictions.
Historical data provides valuable insights into the behavior of cryptocurrencies, capturing market dynamics and the impact of various factors over time. By incorporating this data into machine learning algorithms, analysts can uncover hidden patterns and trends that might not be apparent through traditional analysis methods. The longer the historical data available, the more comprehensive the understanding of cryptocurrency market behavior can be.
Moreover, historical data acts as a benchmark for evaluating the accuracy and reliability of prediction models. By comparing the predicted outcomes against actual market movements, analysts can assess the effectiveness of their models and make adjustments as needed.
In summary, historical data plays a fundamental role in cryptocurrency prediction by providing the necessary information to train machine learning models and uncover patterns that can lead to accurate predictions. It serves as a valuable resource for understanding market dynamics and improving the effectiveness of prediction models in the dynamic and evolving world of cryptocurrencies.
Common Machine Learning Techniques for Cryptocurrency Prediction
There are several common machine learning techniques employed in cryptocurrency prediction that have proven to be effective. One such technique is supervised learning, where historical data with labeled outcomes (such as price movements) is used to train models. These models can then make predictions on unseen data by recognizing patterns and relationships.
Another technique is time series analysis, which focuses on analyzing and forecasting data points in sequential order. Time series models, such as Autoregressive Integrated Moving Average (ARIMA) and recurrent neural networks (RNNs), are commonly used to capture temporal dependencies and predict future cryptocurrency prices based on historical trends.
Furthermore, ensemble methods like random forests and gradient boosting are frequently employed. These methods combine multiple models to improve prediction accuracy by aggregating their individual predictions.
Additionally, deep learning techniques, particularly convolutional neural networks (CNNs) and long short-term memory (LSTM) networks, have gained prominence. These models excel at capturing complex patterns in cryptocurrency data and have shown promise in predicting price movements.
Ultimately, the choice of machine learning technique depends on the specific characteristics of the cryptocurrency data and the prediction task at hand. It is important to consider the strengths and limitations of each technique while selecting the most appropriate one for accurate and reliable cryptocurrency prediction.
Feature Selection and Data Preprocessing in Cryptocurrency Analysis
Feature selection and data preprocessing are crucial steps in cryptocurrency analysis, as they contribute to the accuracy and efficiency of machine learning models. Feature selection involves identifying the most relevant variables or attributes from the available data that can significantly impact the prediction task. By selecting informative features, the model’s complexity is reduced, improving its performance and interpretability.
Data preprocessing is equally important, as raw cryptocurrency data often contains noise, missing values, or inconsistencies. Preprocessing techniques include data cleaning, normalization, and scaling to ensure the data is in a suitable format for analysis. Missing values can be imputed using various methods, and outliers can be handled to prevent them from adversely affecting the model’s training process.
Additionally, feature engineering techniques may be employed to create new features from existing ones, capturing additional information that enhances the predictive power of the model. This can involve transforming variables, creating interaction terms, or incorporating domain-specific knowledge.
By carefully selecting relevant features and preprocessing the data appropriately, analysts can improve the quality and reliability of cryptocurrency analysis. These steps enable machine learning models to better understand the underlying patterns and relationships within the data, leading to more accurate predictions and valuable insights in the dynamic cryptocurrency market.
Overfitting and Generalization Challenges in Cryptocurrency Prediction Models
Overfitting and generalization challenges pose significant obstacles in developing robust cryptocurrency prediction models. Overfitting occurs when a model becomes excessively complex and starts memorizing the training data instead of learning meaningful patterns. As a result, the model may perform exceptionally well on the training data but fail to generalize to new, unseen data.
In cryptocurrency prediction, overfitting can occur due to the high volatility and noise inherent in the market. Models that capture every minute fluctuation may not accurately represent the underlying trends and patterns.
To address overfitting, regularization techniques like L1 and L2 regularization can be employed to penalize overly complex models. Additionally, feature selection, data augmentation, and increasing the size of the training dataset can help combat overfitting by providing a more representative sample of the cryptocurrency market.
Furthermore, model evaluation on validation and test datasets is crucial to assess generalization. If a model performs well on unseen data, it demonstrates its ability to generalize and make accurate predictions beyond the training data.
By mitigating overfitting and achieving good generalization, cryptocurrency prediction models can offer reliable insights and predictions that are applicable to real-world trading scenarios.
Real-World Applications of Machine Learning in Cryptocurrency Prediction
Machine learning has found numerous real-world applications in cryptocurrency prediction, revolutionizing the way investors and traders analyze and forecast market trends. One notable application is Forvest, a leading financial technology company that leverages machine learning algorithms to provide accurate cryptocurrency predictions. Forvest’s Blog serves as a valuable resource, sharing insights and analysis on the intersection of machine learning and cryptocurrency.
By utilizing machine learning techniques, Forvest and similar companies can analyze vast amounts of historical data, including price movements, trading volumes, and market sentiment, to identify patterns and make informed predictions. These predictions can assist investors in making well-informed decisions, optimizing their trading strategies, and managing risks in the volatile cryptocurrency market.
Machine learning in cryptocurrency prediction also enables the development of automated trading systems that can execute trades based on predefined algorithms. These systems can react swiftly to market changes, leveraging the power of machine learning to identify profitable trading opportunities and maximize returns.
Overall, the application of machine learning in cryptocurrency prediction, as exemplified by Forvest’s Blog, offers tangible benefits in terms of accurate predictions, optimized trading strategies, and informed decision-making in the dynamic and fast-paced world of cryptocurrencies.
Rating of this post
Rate
If you enjoyed this article, please rate it.